Financial Regulation and Model Governance since the Financial Crash
August 9th marked precisely a decade since the start of the financial crisis. On that date in 2007, BNP Paribas was the first major bank to acknowledge the risk of exposure to the sub-prime mortgage market when it announced that it was terminating activity in three hedge funds that specialised in US mortgage debt. Inevitably, the regulatory progress as a result has evolved and became extremely rigorous.
Looking back, we can attribute the financial crisis in part to model complexity and systemic obfuscation in the derivatives and credit markets. Banks have been placed under a microscope and scrutinised for their resilience to a wide range of risks. This is hardly surprising, given the post-Global Financial Crisis (GFC) realisation of interconnectedness of systemic risk and the financial services industry, particularly for so-called globally Systemically Important Financial Institutions (SIFIs).
To maintain the industry’s public good of wealth creation, liquidity provision and sound money management, financial services must address deficiencies in model governance by learning from practices implemented by “high integrity” aerospace, medical, and automotive industries. While model and data governance have been elevated in regulations such as TRIM, BCBS 239, Solvency II and the PRA Stress Test guidance, regulators and all industry participants on sell- and buy-sides must work harder to drive thorough model governance standards.
Now, the financial services industry is complex and rightly thrives on complexity – that’s how it fosters wealth creation in the real economy. The industry can manage complexity in risk better, but not by patching together systems with additional spreadsheets and tools of often unknown origin. It can work towards a harmonised architecture/platform which manages, models and reports risk whatever the department and job role, whether a chief risk officer, risk modeller, developer or front office representative. It should be able to deal, too, with the uncoordinated barrage from regulators and supervisors.
In software terms, this is achievable. It has been realised in non-financial organisations. Look at the automotive industry: building an automated safety-first model to production process, with validation and verification, has increased vehicle reliability, assurance and environmental protection, as well as unleashing the vehicle design creativity resulting in multiple new features at reduced cost, and significantly improving the driver experience. This process has enabled the development of safety-first assisted or fully-automated driving delivery of capabilities to the market.
Financial services can and should strive to do the same. It is quite possible for risk, projection and valuation models to be built, customised and improved, rapidly, consistently and in coordination. It is also possible to implement while minimising technical debt, applying good development processes that in turn foster continuous system improvement. Those models can be made available to whoever needs them, whether ardent researcher, FATCA-liable executive or prospective customer.
However, this requires cultural change. Established bureaucracies need to be at worst crushed, at best, reformed. Cries of “we’ve always done it this way” should be challenged. Financial institutions must seek to reduce complexity where complexity adds nothing, both in communication and in model development.
That said, we are seeing an increase in the development of new financial models. After the crash, experts highlighted the importance of model review, or as some presenters termed it, challenger modelling. Regulations are more baked here, with CCAR promoting “benchmark or challenger models”, and SR11-7 favouring “benchmarking”. The Bank of England PRA, has previously pinpointed model review and challenge as processes needing improvement.
Some suggested challenger models could incorporate machine learning, perhaps too black box for current frontline regulatory calculations, but this could prove interesting for validation and potentially improving accuracy.
In comparison to other industries, the financial services industry lacks a certain degree of understanding of software verification – that the software, as opposed to the model, delivers true output. The aerospace industry boasts regulations such as DO178C, which go far beyond anything in the financial services.
But, new financial models have greater predictive capacity and have far superior accuracy overall than previously obtained with linear models. Using an effective mathematical modelling tool, it may be possible to predict another financial crisis before it arrives.
With all this in mind, to succeed in addressing good model governance, financial institutions should aspire to a unified system with reduced operational, model and legal risks, servicing multiple disconnected supervisory regimes, in turn improving productivity through risk-aware development.
A decade on, regulators and banks have tackled the problems of model complexity and systemic obfuscation in the derivatives and credit markets, but the industry now heads into a new bubble of artificial intelligence, even bigger data, crypto-currencies, robo-advisors and a proliferating patchwork of confusing, unsourced and often poorly-supported computer languages, putting the international population at risk of experiencing new global financial and economic crises.
With the wave of new technology, cybersecurity is another factor that must be considered when calculating financial risk. Details of measuring it and bank mitigation are still vague but it’s importance should not overshadow the ongoing problem of human errors, which have the potential to cause an equal amount of damage.
Given that we are still feeling the ramifications of the previous financial crisis, it is imperative that good model governance standards are agreed upon. In this regard, financial risk managers can and should lead the industry in developing sound model governance practices.
About Stuart Kozola
Stuart Kozola is Product Manager Computational Finance and FinTech at MathWorks. He is interested in the adoption of model-led technology in financial services, working with quants, modellers, developers and business stakeholders on understanding and changing their research to production workflows on the buy-side, sell-side, front office, middle office, insurance, and more.
Website: https://uk.mathworks.com/
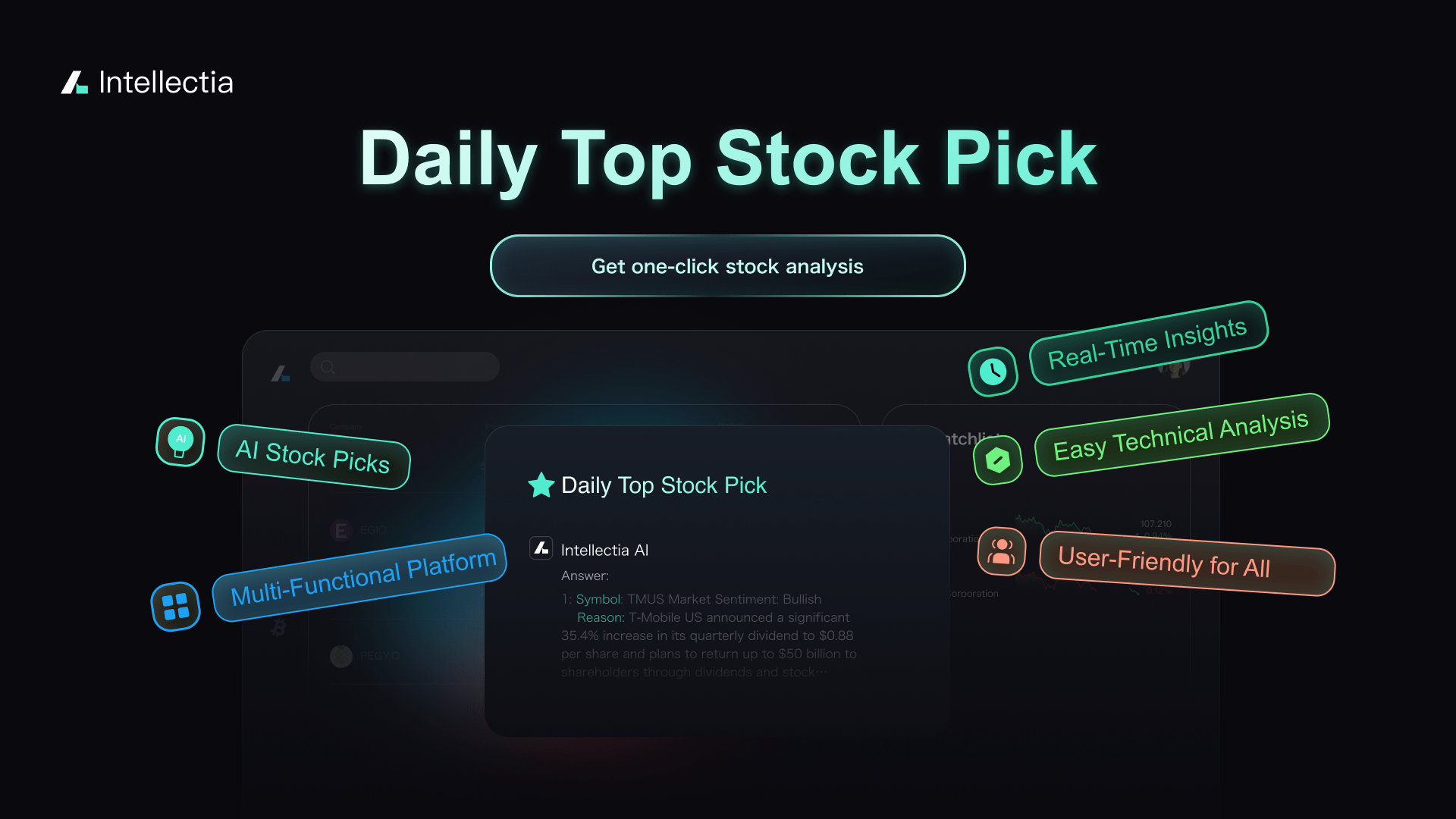