Can AI Help Insurers Detect Cybersecurity Risks?
When it comes to cyber security, we either think of someone stealing our identity via our online presence or the Hollywood style military takeover of an opposing force’s computer network.
The current climate has led more individuals, businesses and government entities to really take a look at what they can do to protect themselves from the very real threat of cyberattacks. Today more than ever, artificial intelligence is playing a larger role in detecting and mitigating cyber risks.
Why do cybersecurity and insurance go hand in hand?
Risk and protection go hand and hand. The more data that is collected on someone or something, the more valuable it can become for someone who wants to use it for malicious intent. Cyber risk is a new type of risk that has appeared in the past 5 years and that is increasing year after year. The attacks themselves can come with little to no warning, and the task of recovering from one is often time-consuming and costly.
Ransomware attacks, distributed denial of service attacks and phishing attacks are just a few of the plethora of ways that attackers can gain access to home and company networks, steal passwords and banking information and go as far as wiping clean the computers in offices, leaving nothing more than a paperweight at each desk. These attacks in fact are so common that 23% of small business owners have had an attack in the last 12 months according to a survey by Hiscox.
Here are some examples of how AI can be used to combat specific types of cyber threats.
1. Data Poisoning
Data poisoning can be seen for literally what it is, taking data and then using it with ill intent. This is done when samples of data that are used for training algorithms are manipulated into having an output or prediction that is hostile that is triggered by specific inputs. This is all the while remaining accurate for all other inputs.
Data Poisoning that turns systems hostile is done before the model training step. Zelros has an Ethical Report standard, where they collect a dataset signature on the successive steps of modelisation. This is a necessary check that needs to be taken that helps prove afterwards that the data has not been tampered with or otherwise manipulated. This standard can be adapted by other companies as one of the best practices when using AI responsibly.
2. Privacy
Entities, whether they be government, law enforcement or even personal networks that have specific features within their dataset that are used to train their algorithm, their identity may be compromised. To avoid an individual or multiple identities being compromised as part of the training data and therefore adding risk to their privacy, organisations can use unique techniques such as federated learning. It boils down to training individual models locally at the source and federating them on a more worldwide scale, to keep the personal data secured locally. In general, it’s good to note that detecting specific samples of outliers and excluding them from the training is a recommended good practise to keep on hand.
3. Bias Bounties
As for older generations of software, sharing the details of an AI algorithm can become more of a liability, especially if it becomes exploited with malicious intent to harm since it provides insights into the model structure and its operation. A countermeasure, brought on by Forrester as a trend for 2022, is bias bounties, which support AI software companies to strengthen and improve their algorithm robustness.
“At least 5 large companies will introduce bias bounties in 2022.”
- According to Forrester: North American Predictions 2022 Guide
Bias bounties are becoming the go-to weapon and armour of defence for ethical and responsible AI because they can help ensure that the algorithm in place is as unbiased and as reliable as possible. All because of the many sets of eyes and different thought processes that review it throughout the course of the campaign.
4. Human Behaviour
Human behaviour can be some of the hardest and easiest to predict. When it comes to data or AI manipulation, our first thought might be malicious activity. However, organisations should stop to reflect on what Personal Data is being willingly shared by people even if it is not knowingly.
Our CyberSecurity main weakness is our ability to propagate knowledge of our identity and activities in seconds to thousands of people. Artificial intelligence or even basic tools that can collect data have given this new behaviour consequences that may prove critical when it comes to cyber security.
Let’s look at an old example for reference, with geo-localisation data that is openly shared on social networks: From 2018, it shows how individual scraps of data can be gathered to provide powerful insights into an individual person’s identity and/or behaviour.
These insights can then actually be used as leveraged by AI systems to categorise ‘potential customer targets’ and provide very specific outputs or recommendations. A more recent reference that can be reviewed is, The Social Dilemma documentary about the world of the “attention economy” that is built on this Personal Data gathering from monumental amounts of information. To decrease the impact and subsequent consequences of our Human behaviour, nothing outperforms culture and scientific awareness. Data Science acculturation is essential for more security of our private data but also for the ethicality that is baked into AI models, as detailed in the first topic of this article.
“AI tools may be too powerful for our own good”: When feeding streams of data on customers, a Machine Learning model may learn much more than we would actually like it to. For example, even when gender is not an explicit data point in customer data, the algorithm can actually learn to infer it through proxy features. All this when a Human could not, at least with that amount of data, in such a limited time. For that reason, analysing and monitoring the ML model is crucial.
To better equips ourselves to anticipate algorithm and model behaviour, and to help prevent from occurring discrimination through proxies, a key element is diversity. This key can be and is often overlooked when discussing AI solutions. Having multiple reviewers that can provide input through their individual cultural, socioeconomic and ethical backgrounds can lower the risks of biases being placed into AI programs. Organisations can also request algorithmic audits by Third parties, which utilise their expertise and workforce diversity if the team themselves lack diversity to complete these tasks themselves.
About the author: Antoine de Langlois is Zelros' data science leader for Responsible AI. Antoine has built a career in IT governance, data and security and now ethical AI. Prior to Zelros he held multiple technology roles at Total Energies and Canon Communications. Today he is a member of Impact AI and HUB France AI. Antoine graduated from CentraleSupelec University, France.
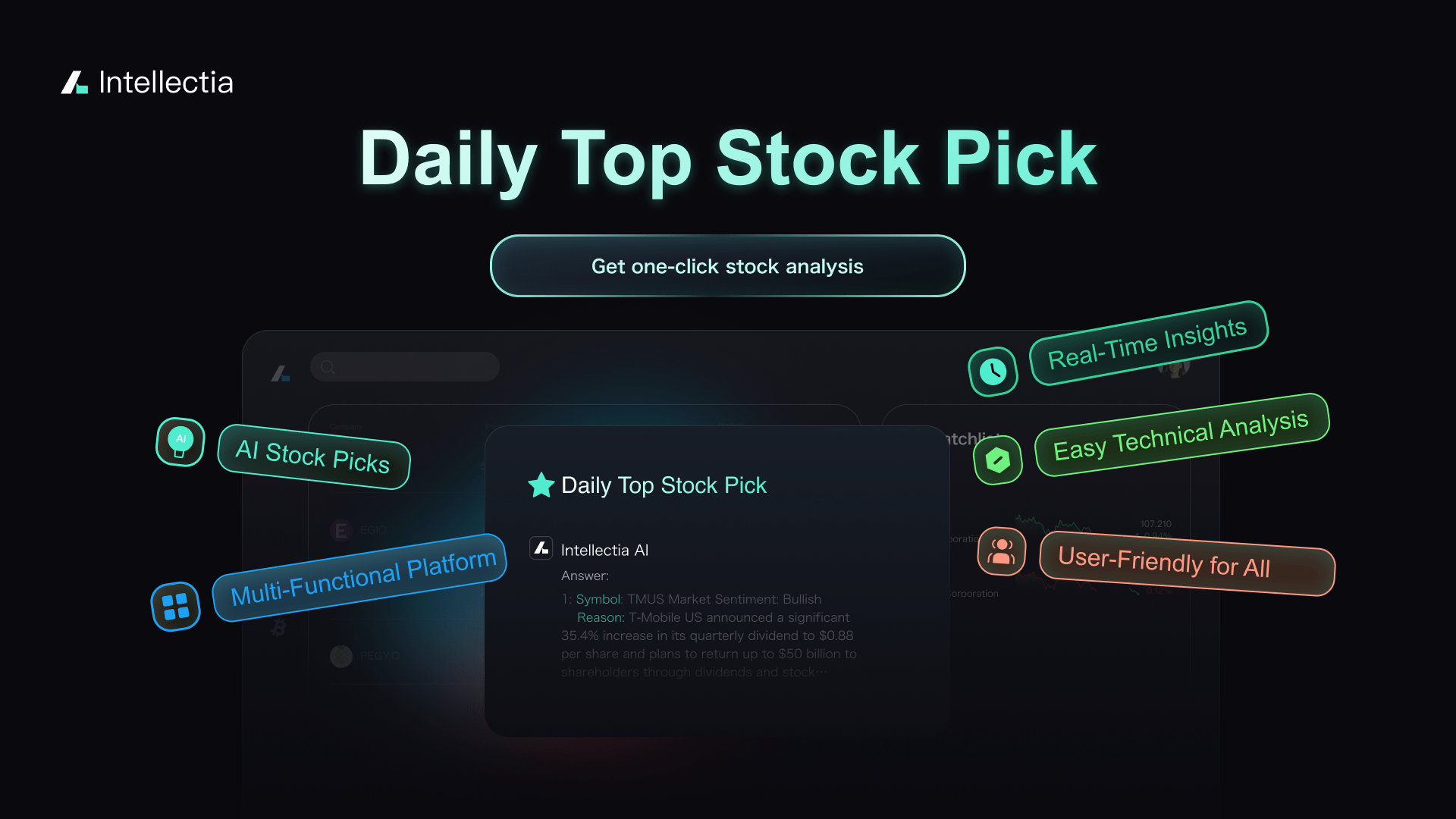