“Data is the new oil.”
You may have heard the words ‘data management’ flying around left, right and centre with no clear understanding on what it is and how paying attention to said meaning could be useful to you, so this month Finance Monthly heard from Maysam Rizvi, a 15-year banking innovator, who provides particular insight into exactly why the data revolution is worth paying attention to. Maysam is the Founder and MD of Aelm, and is responsible for managing change initiatives at international institutions including J.P. Morgan and National Bank of Dubai.
In 2006, UK mathematician Clive Humby coined a phrase that was utterly obvious, hugely prophetic and unerringly timeless. Pointing at the raw material with which we'll build life's next phase, he said: “Data is the new oil.”
In 2017, some 2.5 quintillion bytes of data are created each day. At this rate, it'll take just three months to double the world's entire existing data stock. So Humby's statement is truer now than it was then: data is every industry's imperative. And that's quintuply true for banking.
If financial institutions want to edge ahead, and stay there, it's time to fully embrace data and its possibilities for the long term.
Financial institutions have been longsighted enough to harvest data, but our putting it to work has been sporadic and disorganised. We've been slow to deploy data in areas like regulation and compliance, and we've probably been over keen, and under-effective, in areas like credit and risk.
To digress slightly, I grew up watching movies like Terminator 2: classic struggles depicting robots (bad) versus humans (good). As a young man, I learned – as many of us did – not to trust a world that's in the hands of Artificial Intelligence (AI).
Whenever machines edge out a human workforce, or Hollywood spawns a new cyber villain, robots' reputations nosedive. But it's important to remember that AI is simply a manifestation of data: sets of numbers, trends and analytics built and programmed to perform tasks.
It's daunting, but today's data is the foundation of tomorrow's AI. And the effectiveness of banks' AI will, as the future of finance draws nearer, separate the wheat from the chaff.
The proposition is this: banking will soon rely incalculably on AI. The bedrock of AI is data. We are in a position to mine and manage rich data now.
If the story of the industrial revolution is one of optimising processes and stripping out costs, the tech revolution has utterly multiplied that paradigm.
Twenty years ago, cars started to, basically, build themselves along production lines. Today, quantum data and real-time machine learning means cars can now drive themselves. That's data in action.
And so is this: a 2013 study by Oxford University’s Carl Frey and Michael Osborne estimates that 47 percent of US jobs may be replaced by robots and automated technology within 20 years. Owing to all the brains required, banking is the kind of high cost industry where an AI coup is inevitable.
Since the ATM, we've given pieces of banking over to machines. From internet banking to intricate trading algorithms, anything that can be handed over to machines has been – and will be.
So, that's the proposition. And we can probably make peace with it. Then comes the practical.
How can banks adapt and ensure a steady transition?
On that, there's no quick answer. Whether it's retail or investment banking, preparing for mass AI means dramatically improving technology infrastructures, and sorting a lot of data.
Aside from what already sits in banks' data vaults – and what data is being crunched this very moment – 2017 will bring more machines, software and apps that'll further swell the data highways. We will probably never hit a data ceiling so I can't overstate the importance of a sound and forward-looking data management strategy now.
Central to that strategy are things like business intelligence: drilling quickly to the truth in your data. Storage: expensive server farms versus the Cloud. And security: Tesco got hacked, TalkTalk got hacked – the threat is very real.
Unfortunately, fix-all, pan-department, off-the-shelf AI systems aren't available. So, automated platforms, AI, robots – call them what you will – need to be mapped, developed, integrated and trained. And this data management strategy can't exist in isolation: banks need to roll it up as part of a wider digital strategy, and as part of an overall business strategy.
For starters, new talent is required to develop, design, deploy, analyse and work with new technologies, while current employees will need to be reskilled for a new reality.
Then there's clients and customers. Institutions that are able to construct and manage efficient, intertwining data flows must find ways to push benefits down the chain.
Like it or not, banking is not a trusted industry. Putting more automation between customers and their money or goals may be a bitter pill to swallow. In addition, the AI push will see certain people nudged out of jobs, so banks must think about payoff.
Customers aren't daft. Facebook, Google, Uber - we wearily trade our data in exchange for what, in the end, are personal, hyper-relevant services. Banks need to, basically, come up with their own 'crystal ball' technology.
Uber knows where you are, before, during and, now, even after your ride. It knows where the driver is; how much you'll pay; what service you require.
Uber has a crystal ball. But all that goes to show is that we're not staring down an impossible task. Banks have power, reach and resource at their disposal so my last point, which might sound laughable after all that, is to try and keep things simple.
A comprehensive data strategy for your bank may include only a dozen key end goals, so start there and work back: there are some great brains out there to help you with the detail.
Banks need to believe in and invest in a future made of data. If you don't, the others will.
In fact, the others are.
If you’re a bank looking at AI solutions, I advise you to consider
Where can you apply AI and how to set it up?
How quickly can you adopt an AI solution?
How to manage your team's transition through this technology upscale;
What do you need to do to your existing infrastructure to make this successful?
Tying business strategy closely with technology strategy;
Taking baby steps, solving one problem at a time;
Building the right partnerships to facilitate the transition.
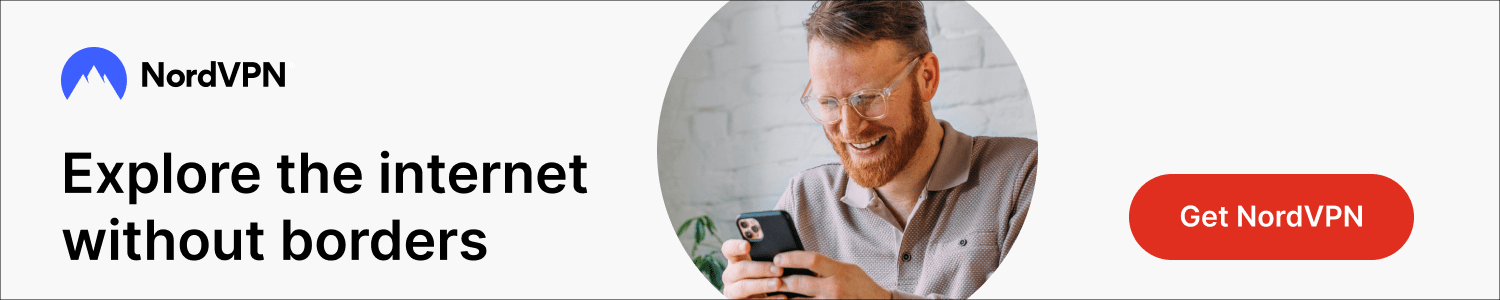