Here’s How Banks Will be Able to Find Out You’ve Committed Fraud
Here Christopher Hillman, Principal Data Scientist at Think Big Analytics, A Teradata Company, delves deep into the processes banks use to identify fraud and the culprits within the system.
Insurance fraud is a growing problem which many insurers have begun to dedicate new departments and whopping budgets to try and tackle. Huge amounts of time and effort is now spent detecting fraud before paying claims to avoid the complexity and expense of recovering a loss – insurance companies certainly don’t want to pay out claims only then to realise they are fake.
Previously, this process involved manually and laboriously going through masses of individual claims while looking out for suspicious activity, creating a large drain on time, revenue and resources. Now, much of that backend research is being completed faster utilising data and analytics, thereby improving the productivity and efficiency of processes while keeping costs down. Despite this, a significant amount of data that might be meaningful never gets analysed and often, advanced analysts still need to be brought in to uncover meaning from results.
Fraud Invaders: a business case
Imagine being able to cut directly to the chase, removing the human effort needed to tackle huge numbers of worksheets to view potentially fraudulent activity. With advanced analytics and visualisation techniques, this is now possible. To demonstrate, let’s look at a business case called Fraud Invaders.
This case aimed to solve an insurer’s crucial business challenge by discovering a new way to focus on a tighter subset of cases to drive fraud investigation efficiency. To begin, claims documents that had been filled out and submitted by the insurer’s customers were collected, some of which were known to be fraudulent. These known cases of fraud were flagged and put through text mining to extract anything that was a clear identifier such as a bank account, email address or phone number. Following this process, analytics were used to uncover correlations between claims.
With this output, a data visualisation (or network graph) was put together. The resulting image, like the one included below, was made up of dots which represent individual claims, with lines which draw data connections between two or more claim documents. An example of a fraud indicator can be monthly insurance payments from the same bank account: chances are the separate claims belong to the same person or are three different people working together to commit fraud.
Not just a pretty picture: how it works
There’s more to see than initially (and appealingly) meets the eye. The dot clusters visible in the image show us who the “fraud invaders” are. The larger and more apparently connected the cluster, the greater the likelihood of fraudulent activity: this ability to gauge the potential for fraud based on the size of dots and amount of connections can be carried out with the need for little more than a quick look.
Using graphs like these as a foundation, claims teams can identify likely suspects and focus their investigations on these groups. Although not all suspects pulled out will turn out to be fraudsters, far less time, revenue and resources will have been required for this process in comparison to traditional, manual methods. In addition, incidents that may have previously slipped through the net may now be uncovered.
Uncapped opportunity: lessons from Fraud Invaders
In addition to helping insurers to identify fraudulent activity, advanced analytics and visualisation can also reveal networks of people and strong influencers who can assist businesses in attracting new customers, or cause them to lose them. This branch of data science, known as “Social Network Analysis” (not to be confused with Social Media) is a powerful technique that requires true multi-genre analytics. A variety of individual techniques are required to produce a model of a customers’ social network including text mining, fuzzy matching, time series processing and graph analytics. By traversing a persons’ network graph, claim teams can see who they are connected with and who they are influenced by when making decisions such as a purchase or switching services.
Overall, regardless of the desired outcome, Fraud Invaders offers a good lesson to businesses in how to achieve what they want: begin with a solution – rather than just a problem – in mind.
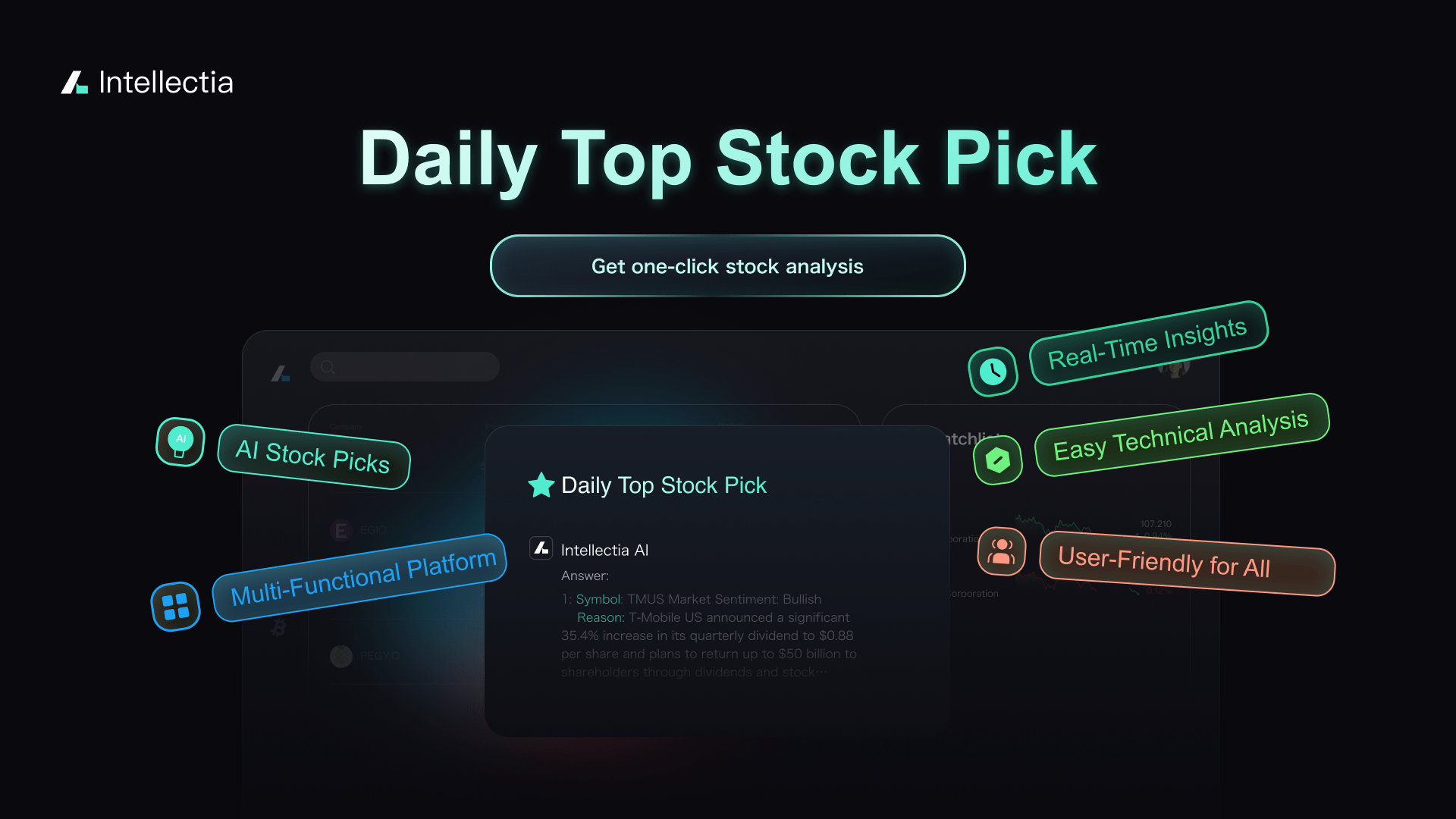